Revolutionizing AI Reasoning: The Tree of Thoughts Breakthrough
Written on
Chapter 1: Understanding the Tree of Thoughts
In a pioneering research paper titled “Tree of Thoughts: Deliberate Problem Solving with Large Language Models,” scholars from Princeton University and Google DeepMind introduced a groundbreaking method that is reshaping how Artificial Intelligence (AI) models reason and tackle complex challenges. The latest iteration, GPT-4, has exhibited an extraordinary enhancement in problem-solving efficiency, achieving a remarkable 900% improvement through this innovative technique known as “Tree of Thoughts” (ToT). This advancement empowers AI systems to make independent and intelligent choices, transcending the limitations of previous prompting methods. This article delves into the origins, methodology, and impressive results of the ToT framework.
Understanding the limitations of traditional prompting methods is crucial before discussing the ToT approach. Traditional input-output prompting (IO) involves posing a question to the AI model and receiving an immediate response. While this technique has had some success, it does not fully exploit the potential of language models.
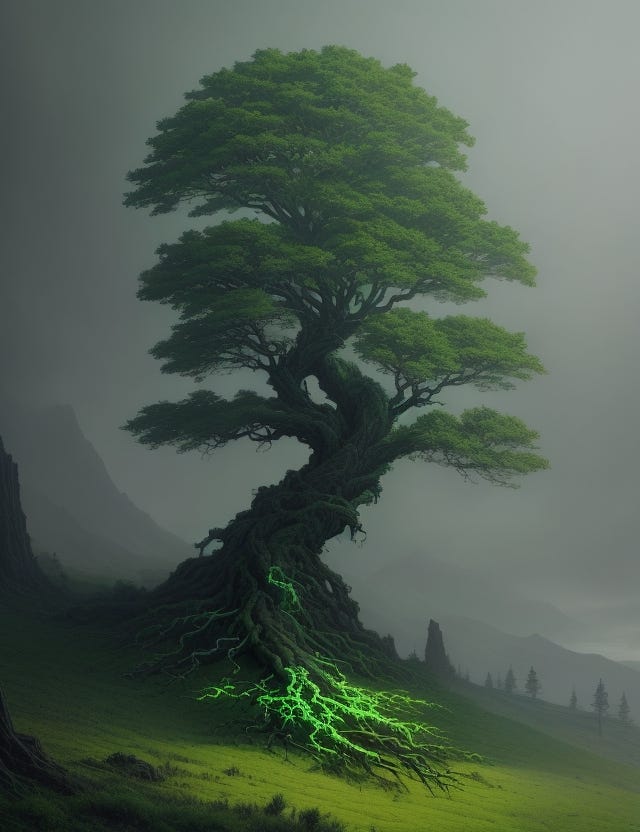
Researchers and practitioners have explored alternative prompting strategies, such as Chain of Thought (CoT), to address these limitations. CoT encourages consideration of various topics or scenarios, selecting the most promising one, and generating responses accordingly. By allowing the model to explore diverse reasoning paths, this method has yielded better outcomes than traditional IO prompting.
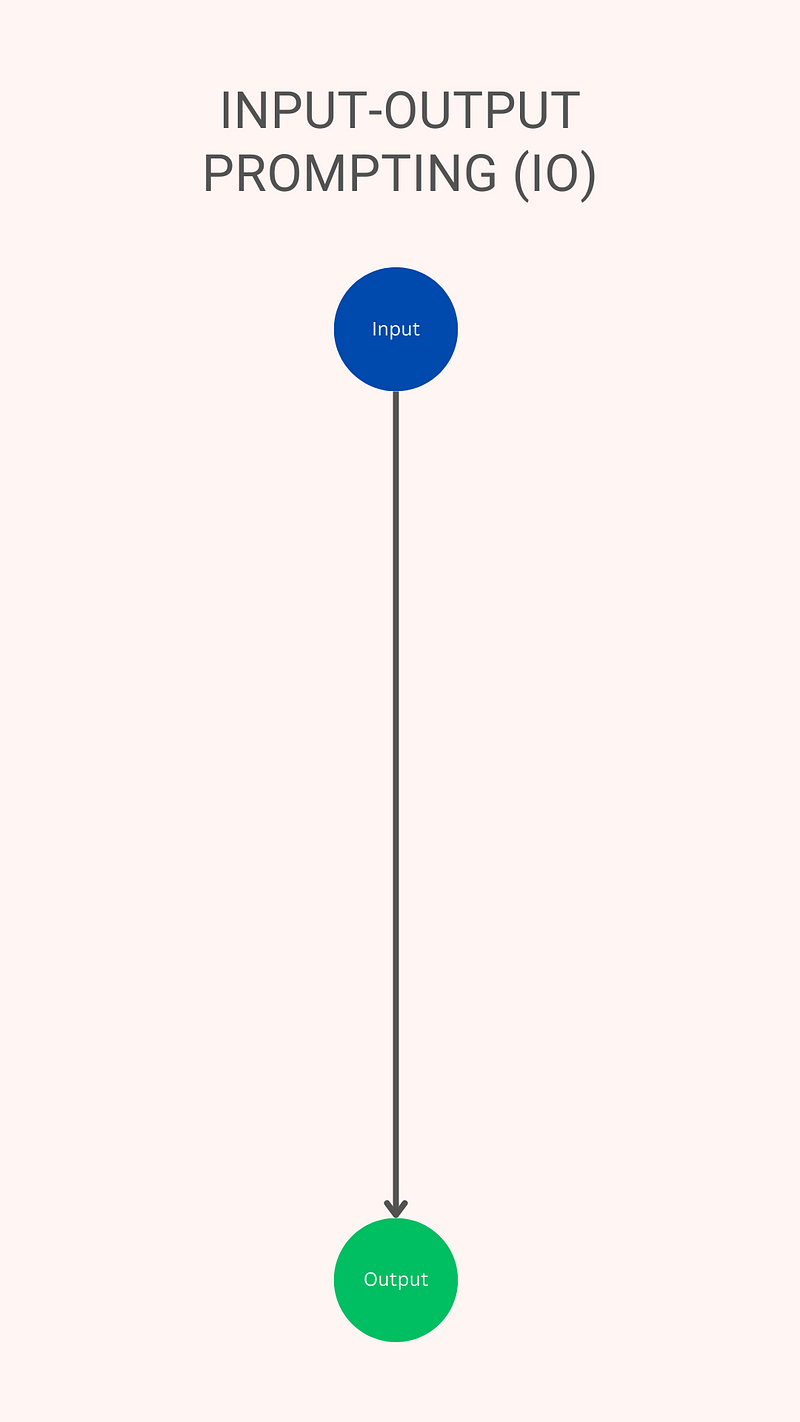
Additionally, a technique known as self-consistency, combined with CoT, has further enhanced results. This method involves generating multiple outputs for each query and selecting the most consistent ones, demonstrating improved performance compared to earlier prompting strategies.
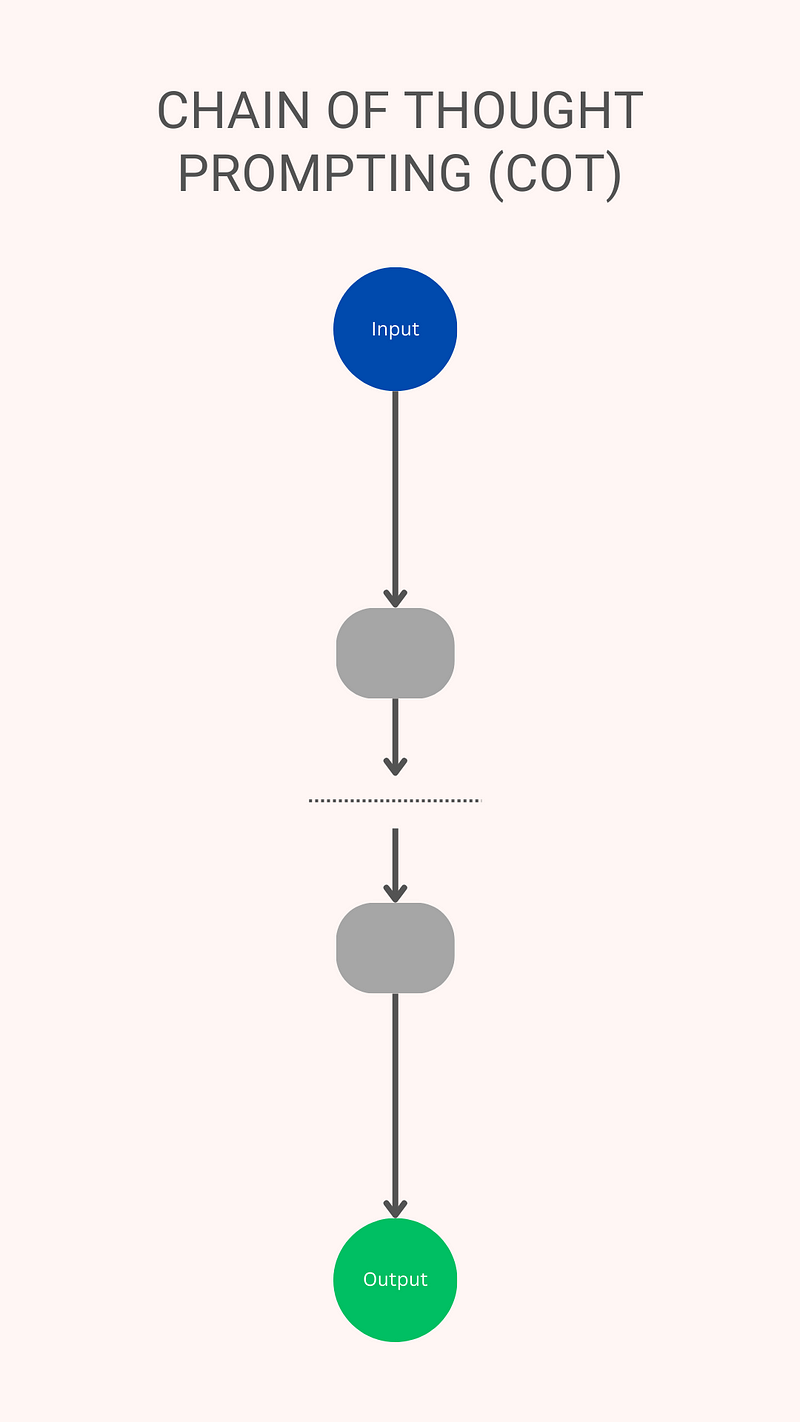
Introducing the Tree of Thoughts Framework
The ToTs framework takes these advancements to a new level, significantly amplifying the capabilities of prior methods. It proposes a paradigm shift in the problem-solving capabilities of language models by introducing a generalized approach that expands upon the CoT concept.
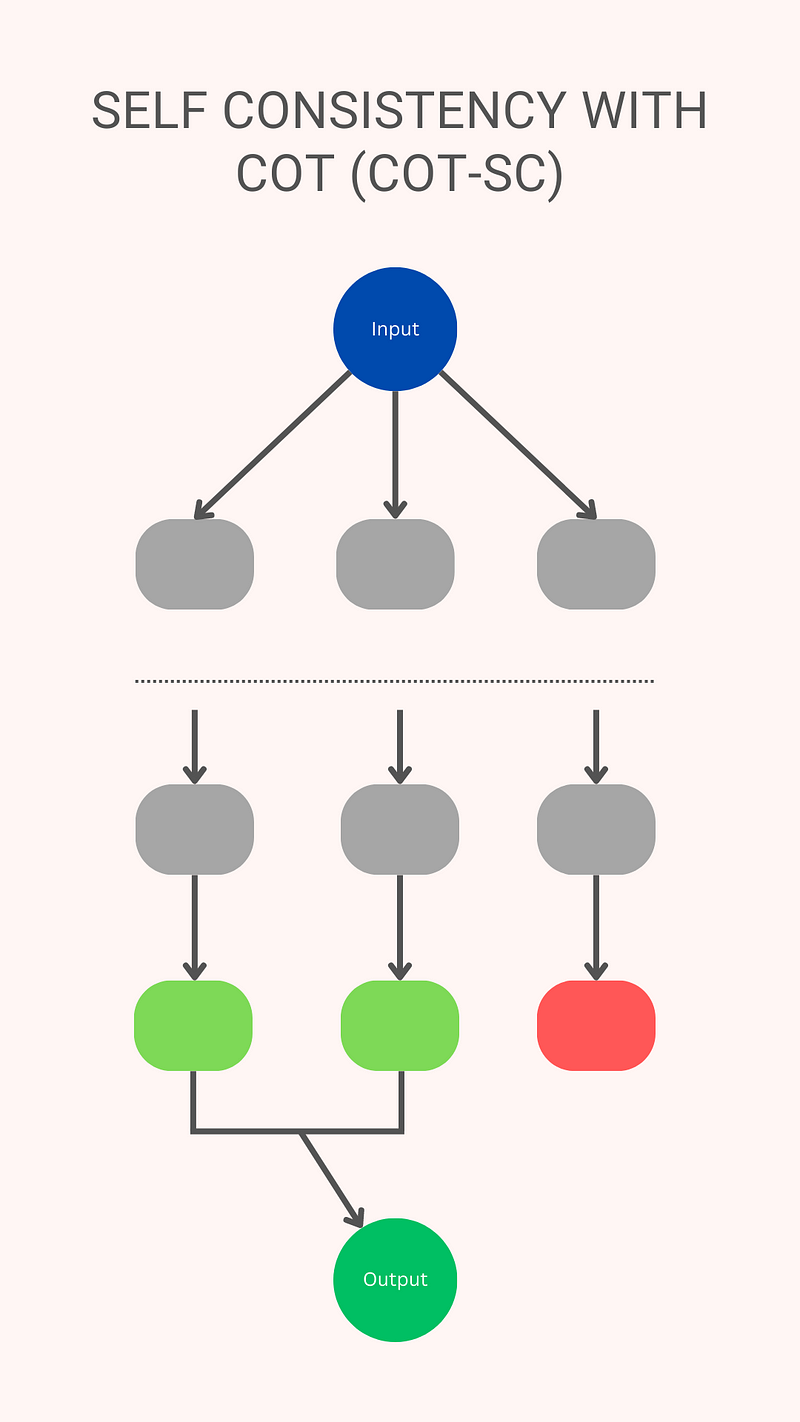
The foundational research conducted by Newell and Simon in 1972, which examined human problem-solving techniques analogous to computer processes, underpins the ToTs framework. Humans explore a multitude of potential solutions, visualizing each as a branch of a tree, with nodes representing partial solutions. By navigating this tree of options through heuristics and rules of thumb, individuals reach answers.
The ToTs methodology empowers language models to investigate various reasoning avenues and make self-assessing decisions, effectively overcoming the limitations of prior prompting techniques. The model’s ability to determine the next step, plan ahead, and backtrack when necessary facilitates more strategic decision-making.
Impressive Results and Applications
Researchers conducted numerous experiments to evaluate GPT-4’s performance across different prompting techniques. One notable test involved the “Game of 24,” a mathematical reasoning challenge akin to Sudoku, where the goal is to achieve 24 using four digits and basic arithmetic operations. The results were astounding: success rates for IO and CoT prompting were 7% and 4%, respectively, while the ToTs method achieved an impressive 74% success rate.
The effectiveness of the ToTs framework was further assessed through creative writing tasks. In this instance, GPT-4 was instructed to generate a coherent four-paragraph text, concluding with one of four randomly provided sentences. Once again, the ToTs approach outperformed others, resulting in a highly cohesive and directive-compliant piece.
Moreover, the researchers explored ToTs in more complex search tasks, such as 5x5 mini-crosswords using natural language cues. The word-level success rates showed significant improvement compared to traditional prompting techniques.
Advantages and Future Implications
While the ToTs framework exhibits remarkable potential, it is crucial to consider both its advantages and limitations. One of its main strengths is enhancing problem-solving capabilities, allowing language models to reason, strategize, and backtrack effectively. The ToT method provides a more comprehensive and informed approach to decision-making by enabling AI models to assess multiple lines of reasoning.
However, employing ToTs requires increased computational resources, which may lead to higher costs. Nevertheless, ongoing open-source initiatives and modular flexibility empower users to adjust performance trade-offs based on their specific needs.
Conclusion
The ToTs paradigm heralds exciting opportunities for AI applications across fields such as education, research, and problem-solving. As language models evolve and integrate the ToT framework, we can anticipate a new era of AI systems demonstrating robust reasoning capabilities, enabling them to tackle complex tasks with unprecedented success rates.
This video provides insights into how the Tree of Thoughts framework significantly improves reasoning capabilities in GPT-4, achieving a 900% enhancement in problem-solving skills.
This video discusses the new prompting technique that leads to a 900% improvement in logic and reasoning in GPT-4, showcasing its transformative potential.
Life is Golden.
— Adam D.
I will receive a little commission if you sign up through my link.
References

This article is published on Generative AI. Connect with us on LinkedIn to receive the latest AI stories and insights directly in your feed. Let’s shape the future of AI together!
