Unveiling 301 New Exoplanets with Machine Learning Techniques
Written on
Chapter 1: Introduction to Exoplanet Discoveries
Recent advancements in machine learning have led to the discovery of an additional 301 exoplanets within the data collected from the Kepler and K2 missions. This remarkable feat was accomplished using a sophisticated deep neural network known as ExoMiner.
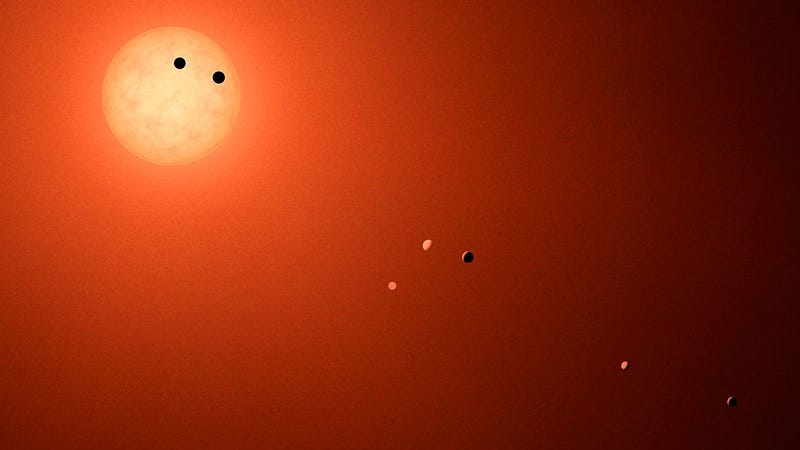
To date, over 4,500 exoplanets have been identified orbiting distant stars, yet it is believed that there are millions more waiting to be discovered within our galaxy. Various techniques exist for detecting these faint celestial bodies, which are often obscured by their larger, brighter stellar counterparts.
Section 1.1: The Role of the Kepler Mission
The Kepler mission continues to enhance our understanding of exoplanetary systems, significantly contributing to the catalog of identified exoplanets. In a recent publication in The Astrophysical Journal, researchers utilized the power of ExoMiner alongside NASA’s Supercomputer Pleiades to expedite the identification and validation of new exoplanets.
Kepler and TESS employ the transit method for exoplanet detection, monitoring variations in a star's brightness to identify potential planets. However, this approach is not without its challenges, as false positives can arise from overlapping star signals, starspots, or even dust. Manually analyzing each star is a time-intensive task, one I can personally attest to.
Subsection 1.1.1: The Impact of Machine Learning
Machine learning offers a solution to these challenges. By training a neural network to distinguish between genuine exoplanets and false positives using previously analyzed data, we can streamline the identification process. With an extensive database of over 4,500 confirmed exoplanets from the Kepler mission, developers successfully trained ExoMiner, achieving impressive results. As project lead Hamed Valizadegan noted, "ExoMiner demonstrates remarkable accuracy and reliability, surpassing both existing machine classifiers and human experts due to inherent biases in human labeling."
While the newly discovered 301 exoplanets are not considered Earth-like or situated within habitable zones, their addition enriches the existing dataset for comparative analysis of exoplanet populations and systems. Kudos to the teams behind Kepler and ExoMiner, including SETI Institute scientists Doug Caldwell and Jeff Smith. We eagerly anticipate their future discoveries.
Chapter 2: Video Insights into Exoplanet Discoveries
In the following section, we explore two insightful videos that delve into the methods and implications of these discoveries.
The first video, titled "The 8th Planet of Kepler-90 - NASA's Discovery Using Machine Learning," provides an overview of how machine learning has facilitated the identification of new exoplanets.
The second video, "Artificial Intelligence and NASA Data Used to Discover Eighth Planet Circling Distant Star," further discusses the role of AI in uncovering exoplanetary systems.
For more detailed insights, please refer to the NASA JPL and USRA press releases, as well as the upcoming article “ExoMiner: A Highly Accurate and Explainable Deep Learning Classifier to Mine Exoplanets” by Hamed Valizadegan and colleagues, set for publication in The Astrophysical Journal.
This content was produced for the Daily Space podcast/YouTube series. For more updates from myself, Dr. Pamela Gay, and Erik Madaus, visit DailySpace.org.